Hey there modern explorer, ready to embark on a quest to unlock the mysteries of Machine Learning and Predictive Analytics? Great! You’ve arrived at the right destination.
In this blog post, we’re going to delve deep into the worlds that surround these buzzwordy topics. Both Machine Learning and Predictive Analytics are pivotal in making sense of the vast pools of the data sea, breathing life into raw, unstructured data and transforming it into meaningful insights. But what exactly do they mean, and how do they differ? Don’t sweat! We’ll untangle these complex threads together!
Two powerful strategies, Machine Learning and Predictive Analytics, are frequently seen locking horns in the arena of data analytics. Yet, they’re unique in their own rights, serving different purposes, utilizing different methodologies, and yielding disparate outcomes.
We’ll start by exploring Machine Learning – what is it, how does it work, where is it applied, and what are its strengths and weaknesses. It’s not magic but pure mathematical sorcery, and we’ll help you decipher it!
Next on our voyage, we brace ourselves to understand the essence of Predictive Analytics. Again, we’re going to walk you through its definition, it’s key elements and operations, real-life applications, and the pros and cons.
Finally, after we’ve equipped ourselves with the understanding of these two powerhouses, we’ll lay them side-by-side and perform a comparative analysis. We’ll shine a light on their key differences, ease of usability, and examine their effectiveness across multiple facets. We’ll also share insights to help you choose the right tool for your business or needs, because let’s admit it, one size doesn’t fit all!
By the end of our journey, you’ll not only have a deep understanding of Machine Learning and Predictive Analytics but also be able to discern where, when, and how to best employ them. So, buckle up! It’s going to be a fun ride!
Stay tuned, keep reading, and let’s unravel these indispensable tools of the data-driven era together. And trust us, this understanding will gear you up for an exciting future in data science!
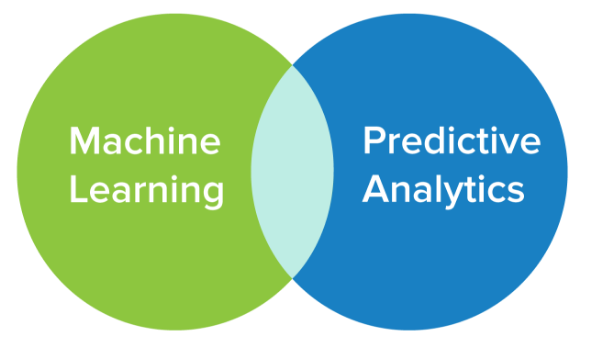
Section 1: Understanding Machine Learning
Definition of Machine Learning
Let’s kick things off by defining machine learning. At its heart, Machine Learning (ML) is a data analysis method. It’s an application of artificial intelligence (AI) that enables computers to learn from experience and adjust to new inputs, all without being explicitly programmed. You might call it a ‘brainy’ computer that can learn patterns, identify trends, and make decisions with minimal human intervention.
Components and Working Principle of Machine Learning
Machine learning isn’t magic, although sometimes it might seem that way. It has key components and follows particular working principles. The main components include the model, the parameters, and the learner.
- The model is the system making predictions or identifications.
- Parameters are the factors the model uses to make these decisions.
- The learner is the component that adjusts the parameters by comparing predictions against reality and learning from the discrepancies.
In essence, machine learning involves feeding data to a generic algorithm. The algorithm then builds its knowledge from the information to make accurate decisions or predictions.
Real-world Applications of Machine Learning
The applications of machine learning are abundant and, to be quite frank, pretty amazing! For instance, machine learning is behind the comfort and easiness of your online shopping experience. It powers the recommendation engines that suggest what book to read next, or which movie you may like. It’s behind the personalized emails you receive from your favorite retailer. It’s even making its way into healthcare, helping predict illnesses and diseases. Yup, machine learning is kind of a big deal!
Pros and Cons of Machine Learning
Everything has its ups and downs, and machine learning is no exception.
On the plus side, machine learning reduces human intervention, makes decisions based on data, not assumptions, and it’s constant learning means that every mistake it makes makes it smarter!
On the other hand, machine learning requires a large amount of data to be effective, can be time-consuming to train, and there’s always the risk that machine learning could end up replacing humans in certain tasks. But hey, no-one said change was ever easy, right?
In the next section, we’ll delve into predictive analytics and see how it compares with machine learning. You’re going to love it! Stay tuned.
Exploring Predictive Analytics
To embrace the full scope of the data revolution, let’s dive into another giant in the field, predictive analytics.
Definition of Predictive Analytics
Let’s simplify. Predictive Analytics, as its name suggests, is all about predictions. Not soothsaying, but diving deep into historical data with complex algorithms and statistical methods to forecast future outcomes. Two essential elements are predictive modelling and forecasting. Rather than reacting to data trends, you’re anticipating them.
Key Elements and Operations of Predictive Analytics
So, how does Predictive Analytics work its magic? Well, it begins its journey with data collection and processing. Without rich and accurate data, the prediction would be akin to blindly phrasing a prophecy.
The second component is the statistical analysis that separates the wheat from the chaff, creating models that make sense of the data. Predictive models then use machine learning or statistical algorithms to churn out forecasts.
And finally, the crucial part – deployment and monitoring of these models. They are then periodically assessed for effectiveness and tweaked to optimise performance.
That said, it’s essential to appreciate that this is a continuous and iterative process. It’s not a “do-it-once-and-forget-it” kind of deal.
Real-world Applications of Predictive Analytics
Predictive analytics isn’t just a fanciful term. It’s very much part of our everyday world. E-commerce companies use predictive analytics to figure out what you might want to buy next. The healthcare industry uses it to predict disease trends and patient outcomes.
Ever wonder how Netflix manages to suggest precisely the kind of shows you’d love to binge-watch next? Yep, you guessed it, predictive analytics! Financial institutions aren’t left out either – they use it to forecast stock market trends and manage risks.
Advantages and Disadvantages of Predictive Analytics
No technology is without its pros and cons, and predictive analytics is no exception. Some of its major advantages are increased efficiency and better decision making. Businesses can leverage future insights to make proactive and informed decisions. However, wrong predictions could lead to severe missteps.
Additionally, their accuracy is heavily dependent on the quality of data. They say garbage in, garbage out. If the data is poor, the prediction will likely not be accurate.
So there you have it—a brief exploration into the world of predictive analytics and its potential benefits. Next, we’ll compare it head-to-head with Machine Learning, so stay tuned!
Machine Learning vs Predictive Analytics: The Main Differences
In simplifying the complexities, let’s illustrate the primal differences between Machine Learning (ML) and Predictive Analytics.
Understanding Machine Learning:
It is a branch of artificial intelligence that empowers systems with the ability to learn and improve from past experiences without being explicitly programmed. Machine Learning prioritizes making predictions based on patterns detected in datasets.
Exploring Predictive Analytics:
On the other side, Predictive Analytics is primarily data-driven. It applies statistical analysis on historical data to forecast future outcomes.
The central difference is that ML systems autonomously improve their efficiency over time, complementing their predictions with constant learning. Predictive Analytics, however, depends on manual re-training to enhance its effectiveness.
Usability: A Comparative Study
When it comes to the ease and usability of both approaches, the value they provide to an organization is determined by the nature of the problem, and the quantity and quality data available.
- Machine Learning: Ideal for creating complex models capable of learning from and adapting to data trends. Its usability is optimum when massive, high-dimensional datasets are available.
- Predictive Analytics: Well-suited for defining and predicting specific outcomes based on existing business data. Mostly useful for targeted forecasting where data dimensions are limited.
Effectiveness: A Side-by-Side Comparison
The effectiveness of Machine Learning and Predictive Analytics is subjective to the array of parameters they process.
- Machine Learning applies a wide range of algorithms, capable of analyzing massive amounts of data to make predictions, thus making it ideal for sectors like healthcare, finance or e-commerce where high volumes of dynamic data are involved.
- Predictive Analytics excels in scenarios where the goal is to predict a specific outcome based on pre-existing data, such as customer churn rate or inventory demand.
Selecting the Right Tool for Your Needs
Your decision to opt for Machine Learning or Predictive Analytics should be guided by the nature and scale of your problem, alongside the volume and depth of data available. Consider Machine Learning when dealing with multi-dimensional data and dynamic environments, while Predictive Analytics would be more beneficial in a smaller, specific forecasting scenario.
Bear in mind, both methods offer distinct, diverse capabilities and may even work together for synergistic advantages in large-scale, data-rich environments.
Conclusion
Subtle yet profound, the subjects of Machine Learning and Predictive Analytics are undoubtedly altering the landscape of technology and business today. As laid out in this comprehensive article, both share the common purpose of deciphering the inherent patterns in data, albeit through different methodologies.
Key Takeaways
- Machine Learning, in essence, is a data processing method that enables systems to learn from data, identify patterns, and make decisions with minimal human intervention.
- On the contrast, Predictive Analytics focuses on utilizing historical data, statistical algorithms and machine learning techniques to identify the likelihood of future outcomes.
Knowing when to use which tool
Selecting between Machine Learning and Predictive Analytics depends largely on your organization’s specific requirements. Short-term forecasting challenges, where identifying future outcomes based on past data is viable, makes Predictive Analytics a superior option. However, when you need to analyze complex data and continuously learn and adapt to new data, Machine Learning emerges victorious.
Envisioning the Future
Peering into the future, we can expect these two fields to grow significantly, possibly in tandem, as they become deeply embedded into the world of Big Data and AI. Machine Learning could continue to form the backbone of AI, evolving to learn even from unstructured data.
Meanwhile, as organizations become more data-driven, the importance of Predictive Analytics is expected to skyrocket, likely aiding businesses to pivot or adapt quickly in volatile markets.
To sum up, whether it’s Machine Learning or Predictive Analytics, the tool is less crucial than the method you use to apply it. Understanding how to harness the power of these technologies effectively is where the real strength lies.
As we close the page on this subject, let’s remember: the goal is to shift from being data-generating to data-powered organizations. After all, the future is data, and Machine Learning and Predictive Analytics are your keys to unlock it.
Stay tuned for more insightful discussions on the evolving world of data analysis technologies!